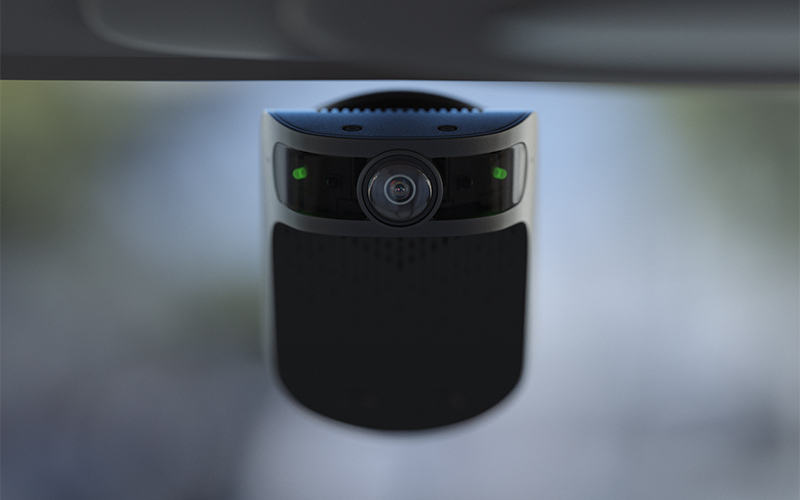
An AI dash cam (dashboard camera) is a single or dual facing camera that is installed into a vehicle to continuously analyze road conditions ahead, and in the case of a dual facing dash cam, also analyze driver behavior, to help drivers reduce distracted driving and avoid collisions by providing driver alerts.
Why fleet and safety leaders are using AI dash cams:
Fleet managers and safety leaders know their greatest resource is their drivers—their ultimate goal is to keep driver’s safe, successful, and feeling valued. However, they also understand how critical it is to keep vehicles and drivers productive on the road, reduce collisions, and decrease liability and maintenance costs. When struggling to balance this, many are turning to AI dash cam solutions to help reduce collisions and costs and keep their driver’s safe.
All AI dash cams are NOT created equal:
More and more fleet safety technology companies are claiming to use artificial intelligence (AI), deep learning, computer vision, and machine learning in their products today. So much so that these terms have become confusing to many. There are many different ways to implement AI—for example, on the network edge (in this case, in the vehicle), in the cloud, or end-to-end from the edge to the cloud. So let’s break these different AI models down and discuss their capabilities.
- On the network edge (in-vehicle) AI processing: AI can be trusted to understand driver behavior, traffic elements, vehicle movement, and critical contextual data across driving ecosystems—but it only works if it can be successfully deployed in the vehicle to help drivers when it matters the most. By running AI-powered algorithms on a device, it enables fleets to understand driver behavior and automatically detect distracted driving and other high-risk behaviors in real-time. Because these AI-powered algorithms run in real-time, you are immediately able to coach drivers with progressive alerts to help them return their focus to the road.
- In the cloud AI processing: Most video telematics and dash cam solutions today require driver video to be uploaded to the cloud for analysis (i.e. for human review) before any distraction determination is made. There are significant shortcomings to this approach because of the time lag, or latency, resulting from data transmission from the vehicle to the cloud and back again which delays real-time alerting. This means the driver doesn’t get a chance to act in time to prevent the incident, and worse, the supervisor knows something has happened even before the driver does (since many systems on the market don’t even let the driver know data was captured).
- Edge-to-cloud implementation: While edge AI processing is purpose-built for real-time collision avoidance systems, Cloud-native software enables rapid iteration for model improvement and offers advantages of high availability, scalability, and reliability. With Nauto, millions of data points from over a billion AI-analyzed video miles are securely stored, meticulously processed, and optimized for driver improvement. From within the cloud, Nauto tests and refines all new and existing convolutional neural network (CNN) model inputs and outputs. This allows us to reach acceptable accuracy levels before deploying over-the-air to create an impact on driver behavior on the edge, in real-time.
What does this mean?
To make the best use of hardware resources on the market today, AI-based driver safety solutions should be implemented across both the edge and the cloud, taking advantage of what each delivers (and does best). This means if your AI Driver and Fleet Safety Platform does not have multi-sensor data fusion, or a multi-tasked convolutional neural network foundation—nor is it optimized for an edge-to-cloud implementation—then it cannot help you predict, prevent, and reduce the occurrence of high-risk events in highly complex driving environments before they happen.
Why Nauto is the leading AI Dash Cam Solution:
Nauto’s dual-facing AI Dash Cams installed in the vehicle’s cabin use computer vision (a form of AI) and proprietary algorithms to assess the situational risk and determine the severity of the distraction, as well as understand potential risks on the road ahead, such as tailgating or nearly running traffic lights and stop signs. The Nauto AI dash cam continuously synthesizes inputs from in and around the vehicle, including driver behavior, vehicle movement, traffic elements, and contextual data, in its multi-tasked Convolutional Neural Networks (CNN) model to determine levels of collision risk. As the detected risk intensifies, Predictive Collision Alerts signals the driver to take action with increasing levels of urgent alerts.